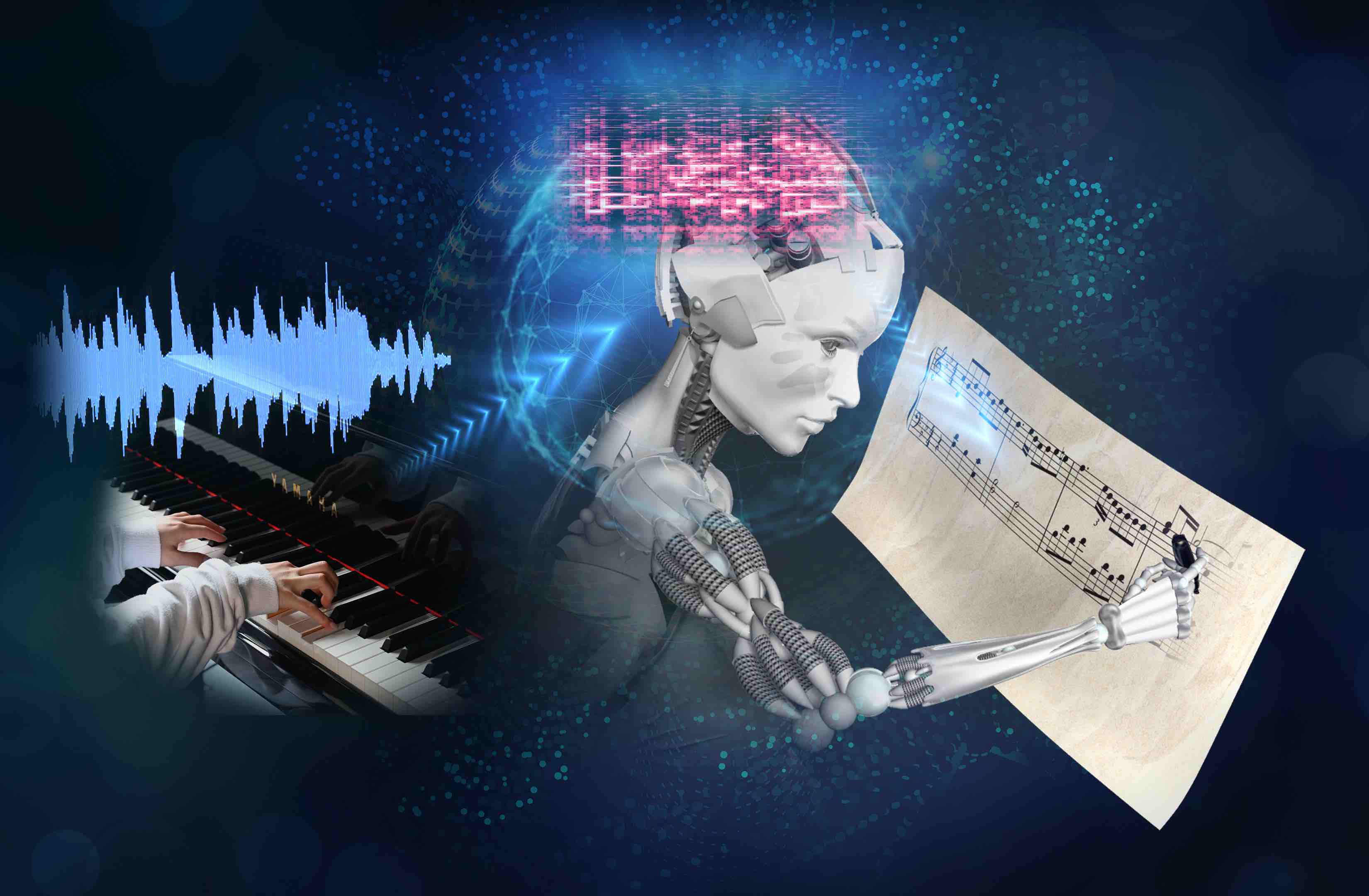
Kentaro Shibata, Eita Nakamura, Kazuyoshi Yoshii
Non-local musical statistics as guides for audio-to-score piano transcription
Information Sciences, Vol. 566, pp. 262-280, 2021. [arXiv:2008.12710]
Non-local musical statistics as guides for audio-to-score piano transcription
Information Sciences, Vol. 566, pp. 262-280, 2021. [arXiv:2008.12710]
Summary of the research
In this paper we investigated a method for generating musical scores from piano performance audio data and achieved a significant improvement in the accuracy by using machine learning techniques.
Music transcription is the task of listening to a music performance and representing it as a musical score; this is a highly intelligent task that only trained experts can do. To transcribe music performances containing multiple tones, such as piano music, it is necessary to recognize complex combinations of pitches and rhythms, and it has been a long-standing difficult problem for researchers to reproduce this ability by a computer. In this study, we realized an automatic transcription system for piano performances by integrating a pitch recognition method using deep learning and a rhythm recognition method using statistical learning, and succeeded in generating high-quality transcribed scores that can partially be used for music performance. We expect that this result will lead to applications for musicological studies and music education, practical systems for assisting musical performance, and a scientific understanding of the intelligence that develops arts and culture. The result also poses a problem of how information technology can affect human culture.
Music transcription is the task of listening to a music performance and representing it as a musical score; this is a highly intelligent task that only trained experts can do. To transcribe music performances containing multiple tones, such as piano music, it is necessary to recognize complex combinations of pitches and rhythms, and it has been a long-standing difficult problem for researchers to reproduce this ability by a computer. In this study, we realized an automatic transcription system for piano performances by integrating a pitch recognition method using deep learning and a rhythm recognition method using statistical learning, and succeeded in generating high-quality transcribed scores that can partially be used for music performance. We expect that this result will lead to applications for musicological studies and music education, practical systems for assisting musical performance, and a scientific understanding of the intelligence that develops arts and culture. The result also poses a problem of how information technology can affect human culture.
Examples of Automatically Transcribed Scores
The following examples are outputs of the POVNet+RQ+NL (or HR+RQ+NL if indicated by *) method.Selected examples
A Whole New World (Alan Menken, cover by Riyandi Kusuma)
Piano medley of hit songs in 2020 (arr. by Misaki Iwamura)*
Butter (BTS, cover by Jichan Park)
Despacito (Luis Fonsi, cover by Jonny May)
Pirates of the Caribbean (Klaus Badelt, cover by Jarrod Radnich)
Demon Slayer (Kimetsu no Yaiba) Medley (cover by Yomi)
Catalogue d'oiseaux (book 1) II. Le loriot (Messiaen, performed by Yvonne Loriod)
Example 50 Piano medley of hit songs in 2020 (arr. by Misaki Iwamura)*
Example 49 Piano medley of J-pop hit songs in the Heisei era (arr. by tjpiano)*
Example 48 Anemone (Goza)*
Example 47 Zonkyou Sanka (Aimer, cover by SLS)*
Example 46 Cry Baby (Official HIGE DANdism, cover by Animenz)*
Example 45 Rain (HARAMI_PIANO)
Example 44 Pale Blue (Kenshi Yonezu, cover by Dentou-san)
Example 43 Butter (BTS, cover by Jichan Park)
Example 42 Despacito (Luis Fonsi, cover by Jonny May)
Example 41 Sun flower (Chinese folk song, performed by Yundi Li)
Example 40 Adagio Cantabile from Pathetique Sonata (Beethoven, performed by Wilhelm Kempff)
Data Download
You can DOWNLOAD the data used in the paper.
The contents of the data are described in README.txt file.
Contact
For any inquiries please contact Eita Nakamura eita.nakamura[at]i.kyoto-u.ac[dot]jpPlease see also other research topics and academic papers.
Tweet
